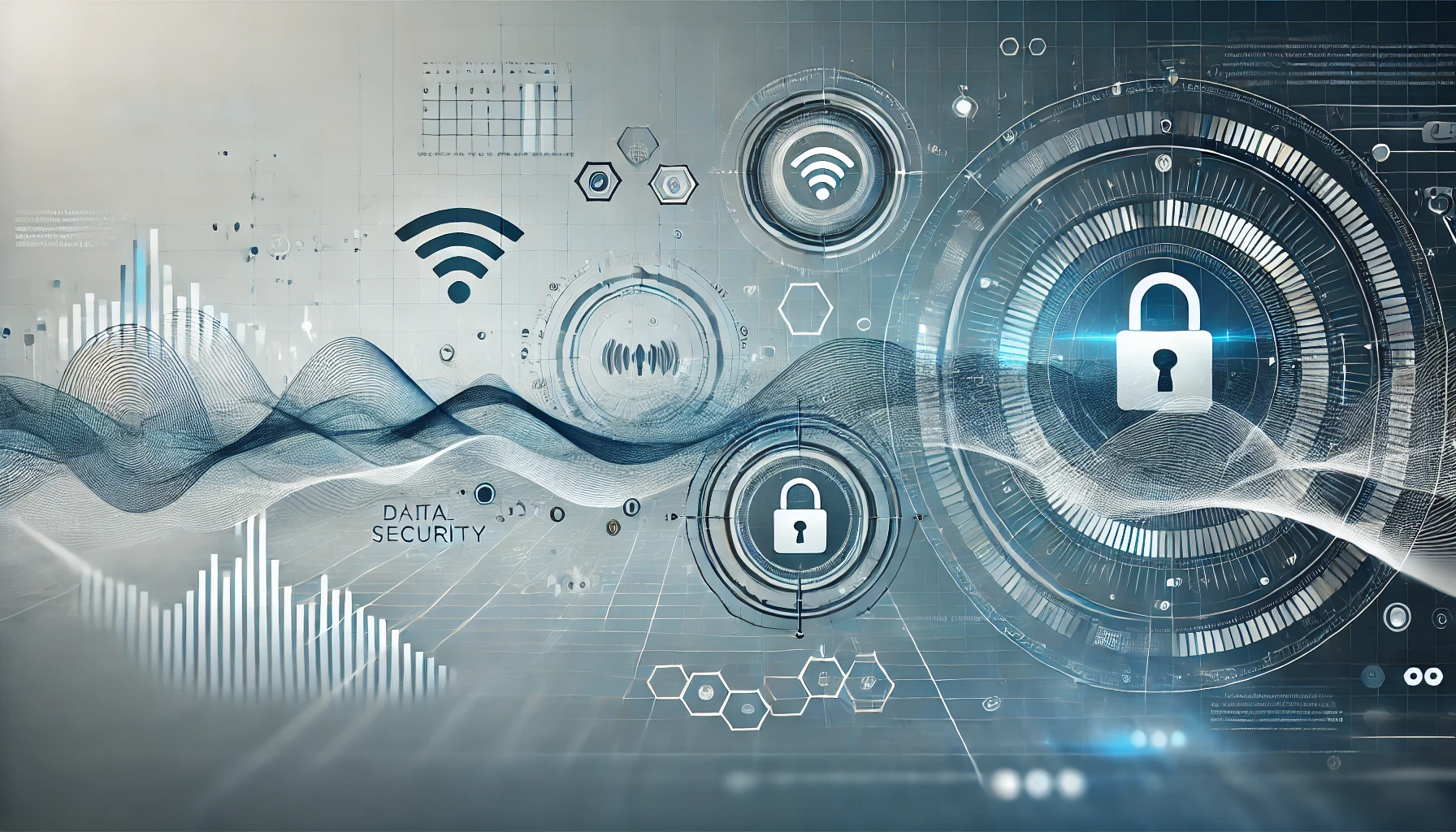
Expedition Technology Receives Phase 3 Data Security Contract from IARPA
Latest Win Continues Multi-Year Effort to Leverage Machine Learning to Protect Secure Information from Compromise in Complex RF Environments HERNDON, VA, July 10, 2024 –
Latest Win Continues Multi-Year Effort to Leverage Machine Learning to Protect Secure Information from Compromise in Complex RF Environments HERNDON, VA, July 10, 2024 –
Company Honored for the Fourth Consecutive Year HERNDON, VA, June 26, 2024 – Expedition Technology (EXP), a leader in the development and deployment of novel
Late last year, Expedition Technology (EXP) achieved another significant milestone in our company’s history – our 100th (and 101st and 102nd) employee joined our team.
If you had to train a GEOINT (geospatial intelligence) object detection algorithm to counter an emerging threat, how quickly could you do it? The past
New Engagement Continues Work Performed for DARPA and Navy on Multi-Phase Radio Frequency Machine Learning System Program HERNDON, VA, November 8, 2023 – Expedition Technology
Milestones present us with natural opportunities for reflection. At Expedition Technology (EXP), we’ve just reached one – our 10th anniversary in business. This achievement simply
And that’s a wrap! The five engineering interns who joined our team at Expedition Technology (EXP) for 10 weeks during the summer of 2023 made
Award Follows Success on Multi-Year, Multi-Phase Knowledge Aided GEOINT Latency Reduction Program HERNDON, VA, September 27, 2023 – Expedition Technology (EXP), a leader in the
Thanks to the dedication and consistent excellence of our fantastic team, Expedition Technology (EXP) continues to rapidly expand. Last summer, we recognized we soon would
Company Will Serve as Subcontractor to L3Harris on Multi-Year, Multi-Phase Engagement HERNDON, VA, June 27, 2023 – Expedition Technology (EXP), a leader in the development
Building teams of great people who explore novel technology and deliver solutions with national impact.
© 2023 – Expedition Technology, Inc., All Rights Reserved