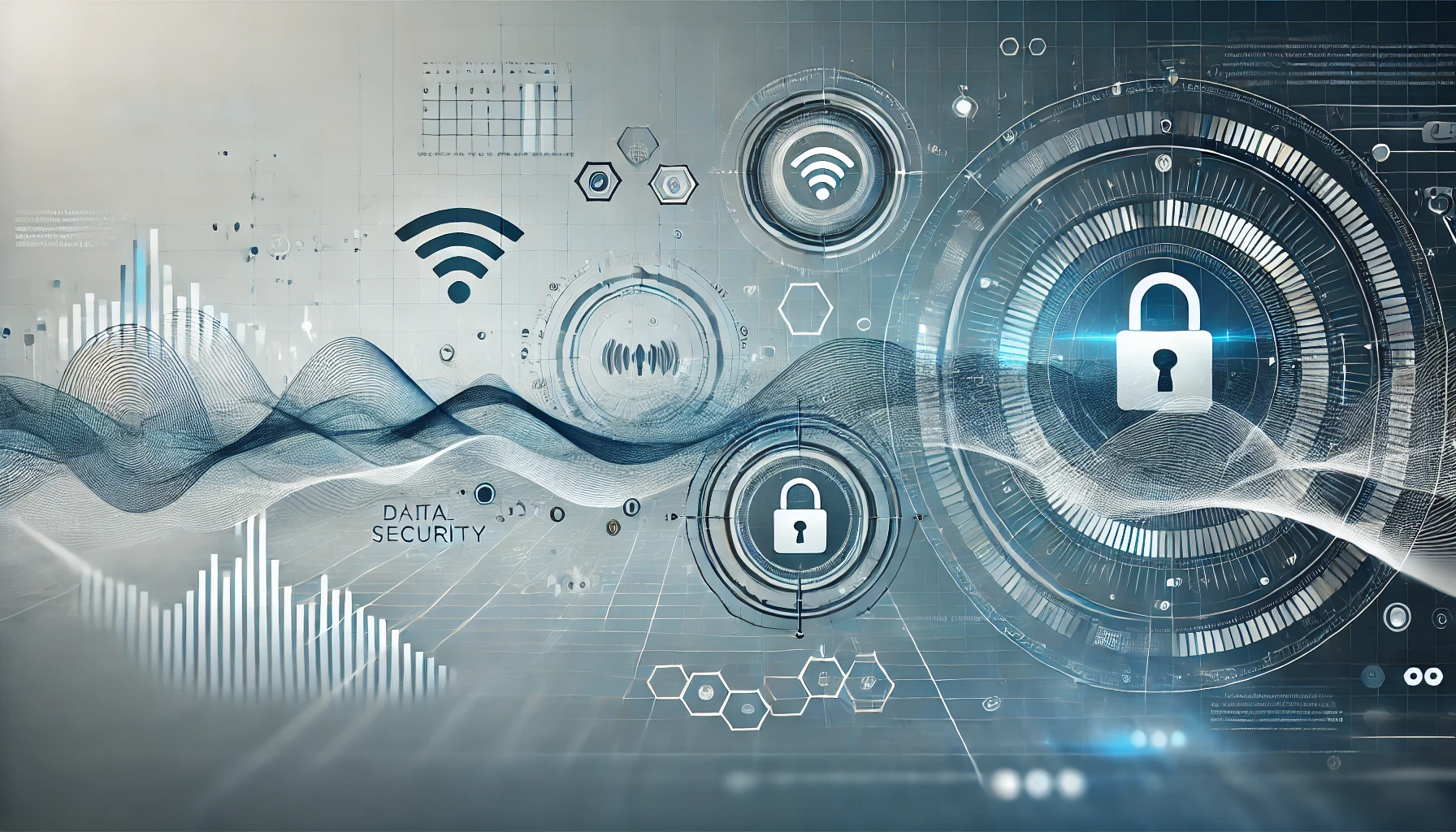
Expedition Technology Receives Phase 3 Data Security Contract from IARPA
Latest Win Continues Multi-Year Effort to Leverage Machine Learning to Protect Secure Information from Compromise in Complex RF Environments HERNDON, VA, July 10, 2024 –
Latest Win Continues Multi-Year Effort to Leverage Machine Learning to Protect Secure Information from Compromise in Complex RF Environments HERNDON, VA, July 10, 2024 –
If you had to train a GEOINT (geospatial intelligence) object detection algorithm to counter an emerging threat, how quickly could you do it? The past
New Engagement Continues Work Performed for DARPA and Navy on Multi-Phase Radio Frequency Machine Learning System Program HERNDON, VA, November 8, 2023 – Expedition Technology
Award Follows Success on Multi-Year, Multi-Phase Knowledge Aided GEOINT Latency Reduction Program HERNDON, VA, September 27, 2023 – Expedition Technology (EXP), a leader in the
When we launched Expedition Technology (EXP) in 2013, our vision of the future was not centered on a specific technology. Building a company that is
by Aimee Moses, Kelsey O’Haire, and Chris Bogart At Expedition Technology (EXP), we ride the cutting edge of research to develop the most impactful solutions
Expedition Technology, Inc. (EXP) announces a Phase 2 Small Business Innovative Research (SBIR) award for continued development of a neural network-based technique to mitigate the effects of
For the past five years Expedition Technology has been developing novel deep learning architectures to solve a broad set of signal processing research problems. While
Expedition Technology, Inc. (EXP) announces a Phase II Small Business Innovation Research (SBIR) award to continue the Improved Geolocation for Over the Horizon Radar (IGOR)
by Michael Person and Carter Brown At Expedition (EXP), we develop state of the art systems for our customers that leverage cutting edge Machine Learning
Building teams of great people who explore novel technology and deliver solutions with national impact.
© 2023 – Expedition Technology, Inc., All Rights Reserved